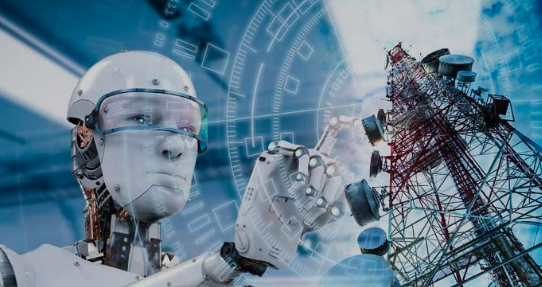
The convergence of Artificial Intelligence (AI) and telecommunication systems marks a paradigm shift in the way communication networks are designed, operated, and optimized. As we delve into the intricate interplay between AI and telecommunication, we embark on a journey to explore the profound impact of AI technologies on network management, performance optimization, cybersecurity, and the overall evolution of communication infrastructure.
Understanding the Nexus: AI and Telecommunication
1. AI in Network Management
a. Intelligent Network Planning
AI empowers telecommunication operators to optimize network planning through predictive modeling and analysis. By considering factors such as traffic patterns, user behavior, and device connectivity, AI algorithms assist in strategically expanding and upgrading network infrastructure.
b. Automated Network Configuration
Traditionally, configuring network parameters has been a manual and time-consuming process. AI automates this task by learning from historical data, ensuring optimal configuration settings for various network elements. This not only enhances efficiency but also minimizes human errors.
c. Predictive Maintenance
AI’s predictive analytics capabilities enable telecommunication companies to anticipate equipment failures and perform proactive maintenance. By analyzing data from network components, AI algorithms can predict potential issues, preventing service disruptions and reducing downtime.
2. AI-Driven Network Optimization
a. Dynamic Resource Allocation
AI algorithms dynamically allocate network resources based on real-time demand, ensuring efficient use of bandwidth and minimizing congestion. This dynamic optimization leads to improved network performance and enhanced user experience.
b. Traffic Management
In congested network scenarios, AI enables intelligent traffic management. By analyzing data patterns, AI algorithms can reroute traffic to less congested paths, optimizing the overall network performance and reducing latency.
c. Quality of Service (QoS) Enhancement
AI plays a pivotal role in enhancing QoS by prioritizing critical services over the network. Real-time analysis of network conditions allows AI systems to allocate resources based on the specific requirements of different applications, ensuring a consistent and reliable user experience.
3. AI in Cybersecurity for Telecommunication
a. Threat Detection and Prevention
Telecommunication networks are prime targets for cyber threats. AI-driven cybersecurity systems analyze network traffic patterns, detect anomalies, and proactively respond to potential security breaches. This real-time threat detection is crucial for safeguarding sensitive data and maintaining network integrity.
b. Behavioral Analytics
AI employs behavioral analytics to identify unusual patterns in user behavior or network activities. By establishing a baseline of normal behavior, AI systems can detect deviations that may indicate malicious activities, enabling timely intervention to prevent cyber threats.
c. Network Security Automation
AI automates security protocols by responding to security incidents in real-time. Automated responses, such as isolating compromised devices or blocking suspicious traffic, enhance the speed and effectiveness of cybersecurity measures.
4. AI and Customer Experience
a. Intelligent Customer Support
AI-powered chatbots and virtual assistants revolutionize customer support in the telecommunication industry. These AI-driven systems provide instant responses to customer queries, troubleshoot issues, and streamline support processes, enhancing overall customer satisfaction.
b. Predictive Analytics for Service Quality
AI analyzes historical data to predict potential service disruptions or quality issues. By proactively addressing these issues, telecommunication providers can improve service quality, reduce customer complaints, and foster greater customer loyalty.
c. Personalized Services
AI algorithms analyze user preferences, behaviors, and usage patterns to offer personalized service recommendations. From tailored data plans to content suggestions, AI enhances the customer experience by delivering services that align with individual preferences.
5. AI in 5G Networks
a. Network Slicing
AI facilitates network slicing in 5G networks, allowing the creation of virtualized, isolated network segments tailored for specific use cases. This enables the coexistence of diverse services with varying requirements on a shared infrastructure.
b. Massive Machine Type Communication (mMTC)
AI supports mMTC in 5G networks by efficiently managing a massive number of connected devices. This is essential for the Internet of Things (IoT) applications, where AI algorithms handle the communication and coordination of a vast array of devices.
c. Ultra-Reliable Low Latency Communication (URLLC)
In URLLC scenarios, such as autonomous vehicles and critical infrastructure, AI optimizes network latency and reliability. By dynamically adjusting network parameters, AI ensures that ultra-reliable and low-latency communication requirements are met.
6. Challenges and Ethical Considerations
a. Data Privacy Concerns
The integration of AI in telecommunication involves the analysis of vast amounts of user data. Ensuring the privacy and security of this data is a paramount challenge, requiring robust measures to protect user information.
b. Bias in AI Algorithms
AI systems are susceptible to biases present in the data they are trained on. In telecommunication, this could manifest as biased service recommendations or discriminatory profiling. Addressing and mitigating biases in AI algorithms is an ongoing challenge.
c. Network Complexity
As telecommunication networks evolve with the integration of 5G and edge computing, the complexity of network management increases. AI systems must navigate this complexity effectively, requiring ongoing advancements in algorithmic efficiency and adaptability.
7. Future Trends: AI and Telecommunication
a. AI-Enabled Edge Computing
The synergy between AI and edge computing will continue to grow. AI algorithms deployed at the network edge will enable faster data processing, reduced latency, and enhanced real-time decision-making.
b. Federated Learning
Federated learning, where AI models are trained across decentralized devices, will gain prominence. This approach allows devices to learn from each other without sharing raw data, preserving user privacy while improving AI model performance.
c. Explainable AI (XAI)
The demand for transparency in AI decision-making will drive the adoption of Explainable AI. In telecommunication, understanding how AI algorithms make decisions is crucial for ensuring accountability and building trust with users.
Conclusion
The integration of AI in telecommunication systems represents a transformative journey toward intelligent, adaptive, and efficient communication networks. From revolutionizing network management to enhancing cybersecurity and elevating the customer experience, AI’s impact is profound and far-reaching. As telecommunication networks continue to evolve with the advent of 5G and beyond, the role of AI becomes even more critical in addressing the challenges and unlocking new possibilities. While navigating ethical considerations and ensuring privacy, the telecommunication industry is poised to harness the full potential of AI, shaping the future of global connectivity.